Livneh gridded precipitation and other meteorological variables for continental US, Mexico and southern Canada
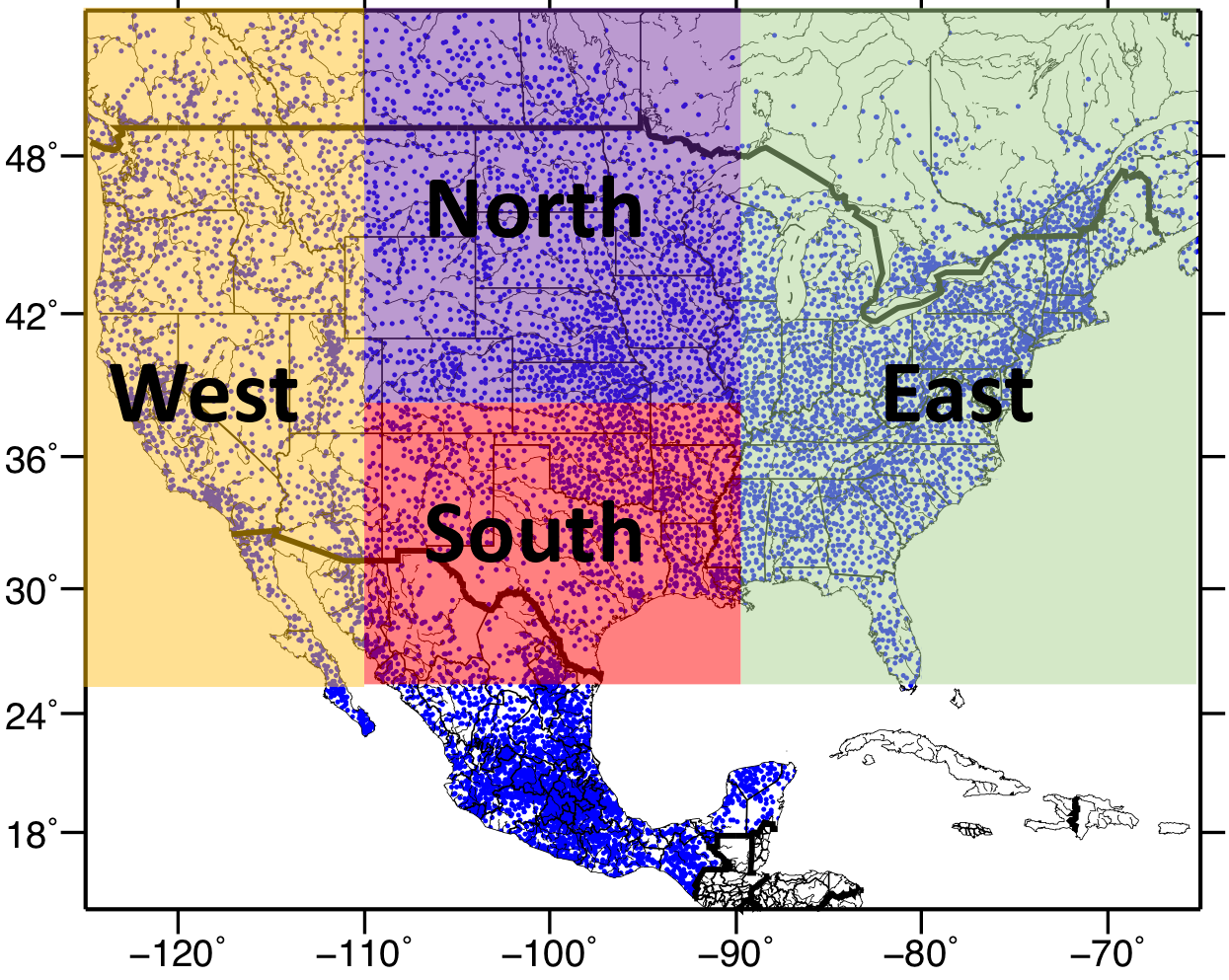
The Livneh et al. (2015) hydrometeorological dataset consists of gridded daily and monthly precipitation, maximum and minimum air temperature, and wind speed for the continental US, southern Canada, and Mexico for the period 1950-2013. Gridded at 1/16° horizontal resolution (~ 6km), the data have been used as training data for GCM downscaling, as validation for numerical weather prediction models, and as the driving datasets for offline land surface models to support a variety of historical investigations. The source temperature and precipitation data are from ~20,000 weather stations in GHCN-daily, Environment Canada, and Servicio Meteorológico Nacional (Mexico). Wind data are downscaled from the NCEP-NCAR Reanalysis.
Key Strengths
Long-term gridded dataset at fine (~6km) horizontal resolution
Inclusive of many stations (~20,000)
Spatially continuous
Key Limitations
Temporal discontinuities; not intended to be used for trend analysis
Relatively simple gridding methodology that requires post-processing to account for topographical effects
The requirement to grid exactly four station values for every grid has the effect of increasing the wet-day frequency of the grids, which can have cascading impacts for derived quantities
Livneh, B., T. J. Bohn, D. W. Pierce, F. Munoz-Arriola, B. Nijssen, R. Vose, D. R. Cayan, and L. Brekke, 2015: A spatially comprehensive, hydrometeorological data set for Mexico, the U.S., and Southern Canada 1950–2013. Scientific Data, 2, https://doi.org/10.1038/sdata.2015.42.
Expert User Guidance
The following was contributed by Dr. Ben Livneh, March 2019:
This dataset represents a station-based daily meteorological product at a 1/16° horizontal resolution (~ 6km) including precipitation, maximum and minimum daily temperature, and wind speed (from reanalysis) for the period 1950-2013, as well as land surface model (LSM) hydrologic output for the same time period. The data were derived from approximately 20,000 stations, with temperature and precipitation lapsed or adjusted for elevation and orographic effects, respectively. The dataset has been used in three general ways. First, as baseline training data for GCM downscaling, serving as the training dataset for the publicly available CMIP5 downscaling (http://loca.ucsd.edu/). The second use has been as validation data for numerical weather prediction models. Third, the meteorological data have been used to drive offline LSMs to support a variety of historical investigations, ranging from flooding to drought, land-cover disturbance, sediment transport, and snowpack simulation. The meteorological data were recently updated (1915-2015) for the CONUS and southern Canada, as well as Mexico (1925-2012). The two primary references are (Livneh et al. 2015; Livneh et al. 2013). The data are in netCDF-3 format, such that no major formatting conversions are needed.
Key strengths of the dataset are: (i) long-term, spanning multiple decades, (ii) inclusive of many stations, requiring a minimum of 20-years of data for stations to be included from CONUS and S. Canada, or 1-year of data for Mexico, and (iii) spatially continuous, with a consistent gridding approach across international boundaries that avoids discontinuities present in other datasets (see Figure 1). Key weaknesses result from: (i) temporal discontinuities that arise from stations coming online or going offline during the period of record, (ii) a relatively simple gridding methodology (the SYMAP algorithm (Shepard 1984)) that requires post-processing to account for topographical effects, and (iii) the requirement to grid exactly four station values for every grid has the effect of increasing the wet-day frequency of the grids, which can have cascading impacts for derived quantities.
The data have been used to attribute the contribution of meteorological versus land-surface moisture states on historical drought (Livneh and Hoerling 2016) and flooding (Badger et al. 2018), as well as to drive LSMs to simulate sediment transport (Stewart et al. 2017) and snowpack evolution (Houle, Livneh, and Kasprzyk 2017). More broadly the meteorological data were used as the training data for a large downscaling effort based on the method of Localized Constructed Analogs (LOCA; (Pierce, Cayan, and Thrasher 2014)), as well as to validate precipitation simulations from numerical weather predictions models (Swales, Alexander, and Hughes 2016; Alexander et al. 2015).
A few key features of the data to aid in interpretation. First, while the gridded product can be interpreted as the mean value over each grid cell, the values are calculated as an interpolation of four stations to the coordinates of the center of the grid cell. Second, and perhaps most important, the time (hour) of observation was used to partition precipitation between days. For example, if a gage station reports precipitation at 6 am, then the methodology splits the 6 am precipitation observation such that 6/24ths is assigned to the current day and 18/24ths assigned to the previous day. This has the effect of underestimating peak daily precipitation values, whereas 3-day or longer compositing periods are largely unaffected. Beyond the aforementioned issues a temperature lapse rate of 6.5 K/km was applied in gridding the station data, which has been shown to produce a cold bias at high elevations in the Pacific Northwest.
An update was made to extend the data through 2015 (e.g. 1915-2015) these data were used in recent papers (Stewart et al. 2017; Marlier et al. 2017). The updated meteorological data can be downloaded here: ftp://livnehpublicstorage.colorado.edu/public/Livneh.2016.Dataset/). An exposition of the updated data through time is provided in Figure 2. This update fixed an issue with the Canadian data, where the distance-weighting of stations was being erroneously impacted by a variable number of digits in the gage ID. Previously the code weighted Canadian stations with shorter station IDs higher than longer ones, primarily affecting grids in Canada and near the Canada/U.S. border in a non-systematic, i.e. random way.
The dataset is methodologically similar to the (Maurer et al. 2002) product, with key distinctions of being higher resolution and longer record length. Other datasets in the same category are NLDAS2 ((Xia et al. 2012); which is shorter and coarser), Daymet ((Thornton et al. 2014); finer, shorter length), the Climate Research Unit (CRU, (Harris et al. 2014); longer, coarser), and the (Newman et al. 2015) product (coarser, shorter, but includes an ensemble). Several papers have compared some of the above products (Livneh et al. 2015; Henn et al. 2018; Lundquist et al. 2015; Ahmadalipour and Moradkhani 2017). Uncertainty has not been formally characterized, although (Livneh et al. 2015) quantify partial uncertainty related to precipitation scaling dataset choice.##
Cite this page
Acknowledgement of any material taken from or knowledge gained from this page is appreciated:
Livneh, Ben & National Center for Atmospheric Research Staff (Eds). Last modified "The Climate Data Guide: Livneh gridded precipitation and other meteorological variables for continental US, Mexico and southern Canada.” Retrieved from https://climatedataguide.ucar.edu/climate-data/livneh-gridded-precipitation-and-other-meteorological-variables-continental-us-mexico on 2025-04-17.
Citation of datasets is separate and should be done according to the data providers' instructions. If known to us, data citation instructions are given in the Data Access section, above.
Acknowledgement of the Climate Data Guide project is also appreciated:
Schneider, D. P., C. Deser, J. Fasullo, and K. E. Trenberth, 2013: Climate Data Guide Spurs Discovery and Understanding. Eos Trans. AGU, 94, 121–122, https://doi.org/10.1002/2013eo130001
Key Figures
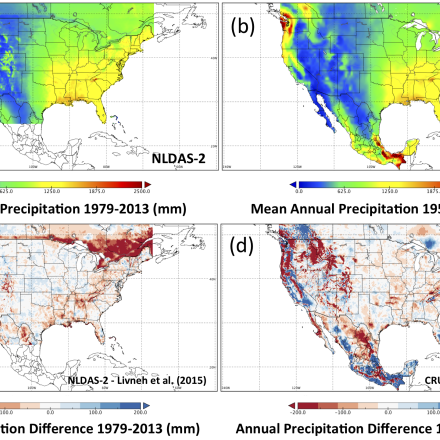
Figure 1: Mean annual precipitation (1979–2013) in (a) and NLDAS2 and (b) CRU v.3.22, as well difference maps NLDAS2 minus Livneh et al. (2015) (c) and CRU minus Livneh et al., 2015, (d). There is a notable transboundary discontinuity in NLDAS2, and NLDAS2 is generally drier than Livneh et al. (2015). Figure adapted from Livneh et al., 2015.(contibuted by B. Livneh)
Other Information
GHCN-daily; Environment Canada; Servicio Meteorológico Nacional (Mexico); NCEP-NCAR Reanalysis
1/16 degree (~6 km)